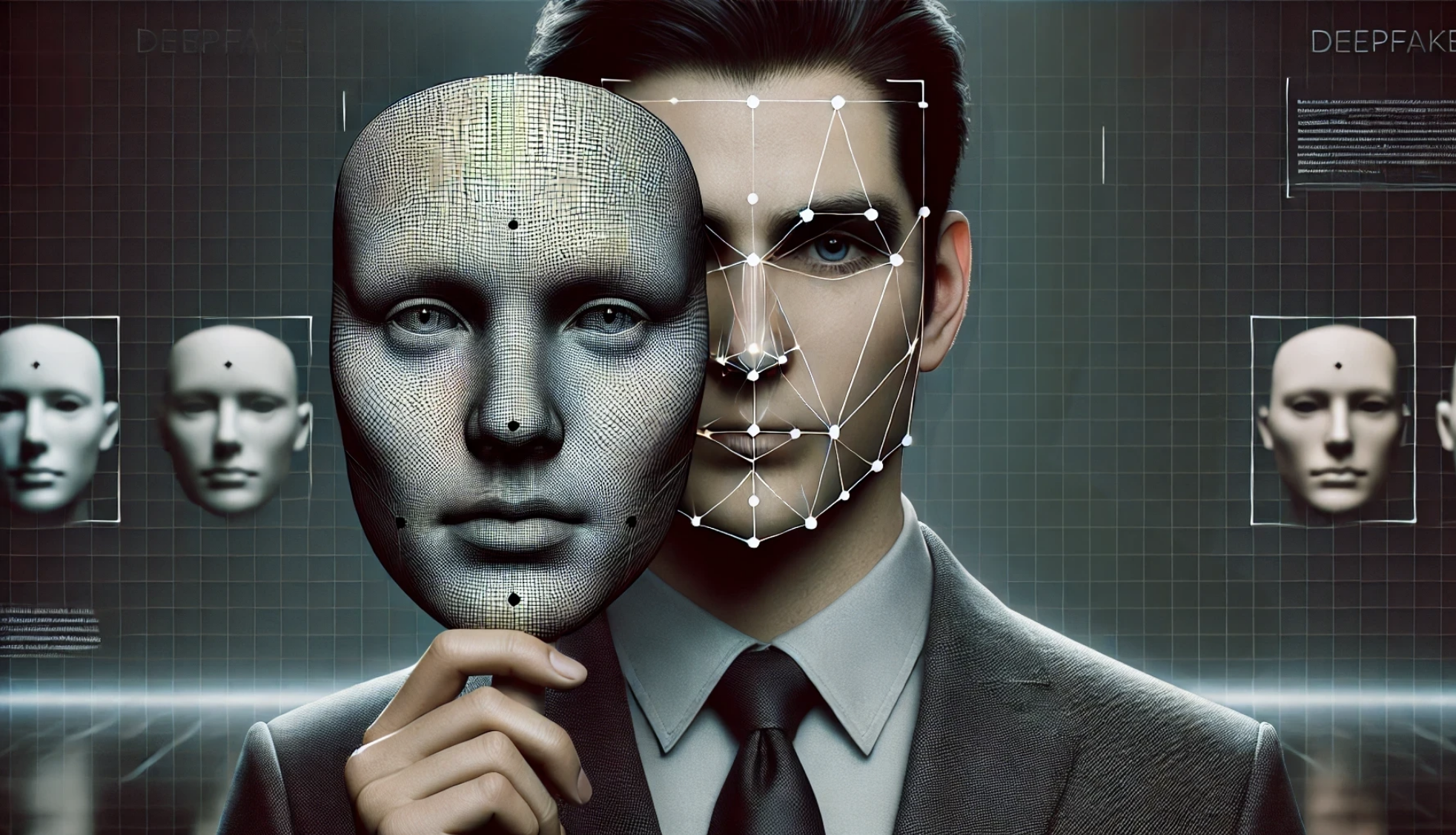
The term “deepfake” has recently flooded mainstream conversations, often accompanied by curiosity and growing concern. While many have encountered deepfake videos, from celebrity impersonations to fabricated political speeches, few people can reliably spot them, and even fewer fully understand how these incredibly realistic fake images and videos are created.
Interestingly, the “deep” in deepfake comes from deep learning, a branch of artificial intelligence that enables computers to analyze vast amounts of data and mimic complex patterns, much like how humans learn from experience. Through deep learning, AI models can generate synthetic media that can be nearly indistinguishable from reality.
The Data and the AI Behind Deepfakes
The process begins with collecting a vast amount of data, which forms the foundation of any convincing deepfake. To replicate a person’s face or voice, creators need a large dataset of images and videos of that individual. These can come from interviews, social media, public appearances, and other publicly available images or footage. The more diverse and high quality this dataset is, including different angles, lighting conditions, and facial expressions, the more lifelike and convincing the result will be. The creation of a realistic deepfake typically requires hundreds, if not thousands, of image and video samples to train AI effectively, according to Scientific American (Firth, 2023). This is one reason why public figures, whose likenesses are widely available online, are common targets.
Once the data is gathered, the next stage involves training a sophisticated AI model known as a neural network. These neural networks are algorithms designed to process and reproduce human-like patterns, such as facial movements and expressions. Two of the most commonly used approaches are generative adversarial networks, known as GANs, and autoencoders.
GAN architecture works through a creative competition between two AI systems: a generator, and a discriminator. Throughout the training process, these two systems challenge each other, the generator creates hyper realistic images as it strives to fool the discriminator. In turn, the discriminator adapts to better identify fake content. This process requires massive volumes of data to work effectively and creators using this technology would face limitations when working with small data sets (Almars 2021).
Autoencoders take a different approach, this procedure involves the training of a neural network to encode and decode images. They learn to compress and then reconstruct images, allowing them to map one person’s facial expressions onto another’s face in a seamless way. These AI models have become remarkably good at capturing even the smallest facial details and subtle expressions, making their output highly convincing, according to The New York Times (Roose, 2019).
After the AI has been trained, the process moves to creating the deepfake itself. A source video is used as the foundation, often a clip of someone speaking or acting. The AI model maps the target person's face onto the individual in the source video. The system automatically adjusts facial expressions, eye movements, and lip synchronization to align with the speech and gestures.
Tools for creating Deepfakes
Some AI tools, such as Wav2Lip, specialize in synchronizing lip movements with speech, making it appear as though the target person is naturally speaking the lines from the source video. These tools generate highly accurate mouth movements even in challenging environments, adding to the realism of the final product. Alongside Wav2Lip, a growing number of deepfake creation tools are now widely accessible, ranging from user-friendly apps to advanced research platforms. DeepSwap, for instance, is popular for casual use due to its speed and simplicity. DeepFace Lab offers more sophisticated capabilities for face swapping and de-aging but requires technical skill to operate effectively. Other tools, such as Deep Nostalgia, animate still images with impressive realism but have raised ethical concerns when used to animate photos of deceased individuals. The increasing availability of these tools reflects how accessible deepfake technology has become, while also highlighting the serious ethical questions it poses (Alanazi & Asif, 2024).
Even after the AI generates the initial deepfake, the work is not complete. Most high-quality deepfakes require careful refinement and editing to ensure that the output is visually seamless. Post-production techniques such as color correction are used to match the artificial face with the lighting and tones of the original scene. Editors also blend edges to make sure there are no visible lines or distortions where the AI-generated face meets the real body and background. Finally, frame smoothing ensures that transitions between frames are fluid and that facial movements appear natural. These refinements are critical for creating a final product that looks and feels authentic, especially when viewed by a discerning audience.
Deepfake detection
As deepfakes become more advanced and widespread, the need for effective detection methods has grown significantly. Early detection approaches focused on identifying visual glitches and inconsistencies such as unnatural facial movements or distorted features. However, many modern deepfakes have become so sophisticated that researchers now rely on deep learning models trained to recognize subtle patterns that differentiate real from fake content. These models require large datasets of authentic and manipulated videos to learn effectively, though a lack of standardized benchmarks still makes it difficult to measure detection tools' accuracy.
Among the more promising methods for detecting deepfakes are those that analyze both visual and temporal inconsistencies in videos. Researchers have explored approaches that detect subtle artifacts like facial warping, unusual eye blinking, and irregular lip synchronization. Others have focused on physiological markers, such as heart rate patterns captured from facial videos, to identify signs of manipulation that may not be perceptible to the human eye. Additionally, distributed ledger technologies are being studied as a way to track the origins of media and verify authenticity. Despite these efforts, keeping pace with rapidly evolving deepfake technology remains an ongoing challenge. As deepfake technology continues to improve, the development of more efficient, adaptable, and accessible detection tools remains a critical area of focus for researchers and policymakers alike (Alanazi & Asif, 2024).

Why Understanding Deepfakes Matters
While this technology is undeniably impressive and holds potential for positive applications, including in film, education, accessibility, and creative arts, it also presents serious risks. One of the most alarming aspects of deepfake technology is its misuse for harmful purposes.
Research from Deeptrace found that 96 percent of all deepfakes found online are nonconsensual pornography, disproportionately targeting women (Deeptrace, 2019). In addition to intimate image abuse, deepfakes have been used in misinformation campaigns, political manipulation, and financial fraud. As these tools become more accessible and easier to use, anyone with a computer and the right software could create a deepfake. This raises urgent concerns about privacy, consent, and the erosion of trust in our digital environments.
Understanding how deepfakes are made is an essential step toward recognizing them and protecting ourselves in an era where seeing is no longer believing. By becoming more informed, we are better equipped to question the authenticity of what we see online and to consider the broader implications of synthetic media. But awareness alone is not enough. Mitigating the risks of deepfakes also requires thoughtful policymaking to regulate harmful uses, public awareness campaigns to educate people about what deepfakes are and how to spot them, and strong collaboration to create effective solutions.
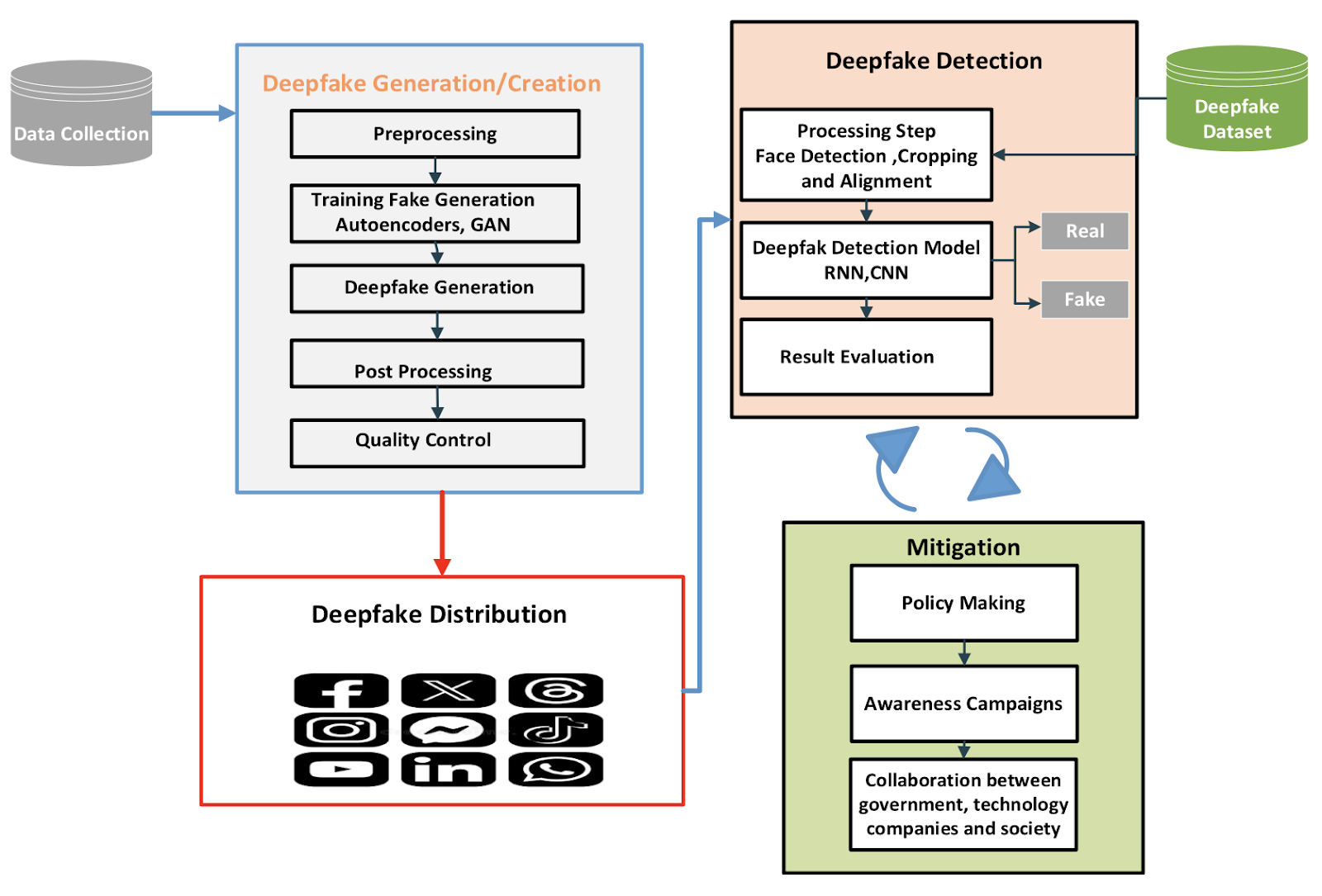
References
Alanazi, S., & Asif, S. (2024). Exploring deepfake technology: Creation, consequences and countermeasures. Human-Intelligent Systems Integration, 6, 49–60. https://doi.org/10.1007/s42454-024-00054-8
Deeptrace. (2019, October). The state of deepfakes: Landscape, threats, and impact. https://regmedia.co.uk/2019/10/07/deepfake_report.pdf
Firth, N. (2023, May 22). Deepfake videos are getting much easier to make. Scientific American. https://www.scientificamerican.com/article/deepfake-videos-are-getting-much-easier-to-make/
Prajwal, K. R., Mukhopadhyay, R., Namboodiri, V. P., & Jawahar, C. V. (2020). A lip sync expert is all you need for speech to lip generation in the wild. ACM Multimedia. https://arxiv.org/abs/2008.10010
Roose, K. (2019, November 21). The making of a deepfake: How to create a convincing fake video. The New York Times. https://www.nytimes.com/2019/11/21/technology/deepfake-video.html